Fundamentals of Statistical Signal Processing
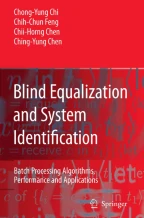
We have reviewed the fundamentals of discrete-time signals and systems, including the definitions, terminologies, transformation tools, and parametric models. We then reviewed random variables and random processes, including statistical characterization, second-order and higher-order statistics for both real and complex cases. Finally, estimation theory was introduced that includes the problem, properties of estimators and several representatives of estimation methods.
This is a preview of subscription content, log in via an institution to check access.
Access this chapter
Subscribe and save
Springer+ Basic
€32.70 /Month
- Get 10 units per month
- Download Article/Chapter or eBook
- 1 Unit = 1 Article or 1 Chapter
- Cancel anytime
Buy Now
Price includes VAT (France)
eBook EUR 42.79 Price includes VAT (France)
Softcover Book EUR 52.74 Price includes VAT (France)
Tax calculation will be finalised at checkout
Purchases are for personal use only
Preview
Similar content being viewed by others
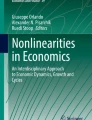
Signal Processing
Chapter © 2021
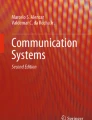
Signal Analysis
Chapter © 2020
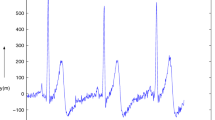
Professor C R Rao’s contributions in statistical signal processing and its long-term implications
Article 25 July 2020
References
- A. V. Oppenheim and R. W. Schafer, Discrete-time Signal Processing. New Jersey: Prentice-Hall, 1999. Google Scholar
- S. J. Orfanidis, Introduction to Signal Processing. New Jersey: Prentice-Hall, 1996. Google Scholar
- O. K. Ersoy, Fourier-related Transforms, Fast Algorithms and Applications. New Jersey: Prentice-Hall, 1997. MATHGoogle Scholar
- S. A. Dianat and M. R. Raghuveer, “Fast algorithms for phase and magnitude reconstruction from bispectra,” Optical Engineering, vol. 29, no. 5, pp. 504–512, May 1990. ArticleGoogle Scholar
- H.-M. Chien, H.-L. Yang, and C.-Y. Chi, “Parametric cumulant based phase estimation of 1-D and 2-D nonminimum phase systems by allpass filtering,” IEEE Trans. Signal Processing, vol. 45, no. 7, pp. 1742–1762, July 1997. ArticleMATHGoogle Scholar
- C.-Y. Chi, “Fourier series based nonminimum phase model for statistical signal processing,” IEEE Trans. Signal Processing, vol. 47, no. 8, pp. 2228–2240, Aug. 1999. ArticleMATHMathSciNetGoogle Scholar
- C.-Y. Chen and C.-Y. Chi, “Nonminimum-phase complex Fourier series based model for statistical signal processing,” in Proc. IEEE Signal Processing Workshop on Higher-Order Statistics, Caesarea, Israel, June 14–16, 1999, pp. 30–33. Google Scholar
- C.-H. Chen, C.-Y. Chi, and C.-Y. Chen, “2-D Fourier series based model for nonminimum-phase linear shift-invariant systems and texture image classification,” IEEE Trans. Signal Processing, vol. 50, no. 4, pp. 945–955, April 2002. ArticleMathSciNetGoogle Scholar
- A. V. Oppenheim and R. W. Schafer, Discrete-time Signal Processing. New Jersey: Prentice-Hall, 1989. MATHGoogle Scholar
- W. A. Gardner, Introduction to Random Processes with Applications to Signals and Systems. New York: McGraw-Hill, 1990. Google Scholar
- A. Papoulis, Probability, Random Variables, and Stochastic Processes. New York: McGraw-Hill, 1991. Google Scholar
- Y. Dodge, Ed., The Oxford Dictionary of Statistical Terms. New York: Oxford University Press, 2003. MATHGoogle Scholar
- P. O. Amblard, M. Gaeta, and J. L. Lacoume, “Statistics for complex variables and signals-Part I: Variables,” Signal Processing, vol. 53, pp. 1–13, 1996. ArticleMATHGoogle Scholar
- E. A. Cornish and R. A. Fisher, “Moments and cumulants in the specification of distributions,” Reviews of the International Statistical Institute, vol. 5, pp. 307–320, 1937. ArticleGoogle Scholar
- J. M. Mendel, “Tutorial on higher-order statistics (spectra) in signal processing and system theory: Theoretical results and some applications,” Proc. IEEE, vol. 79, no. 3, pp. 278–305, Mar. 1991. ArticleGoogle Scholar
- D. R. Brillinger, Time Series: Data Analysis and Theory. New York: McGraw-Hill, 1981. MATHGoogle Scholar
- J. M. Mendel, Lessons in Estimation Theory for Signal Processing, Communications, and Control. New Jersey: Prentice-Hall, 1995. MATHGoogle Scholar
- C. L. Nikias and A. P. Petropulu, Higher-order Spectra Analysis: A Nonlinear Signal Processing Framework. New Jersey: Prentice-Hall, 1993. MATHGoogle Scholar
- C. L. Nikias and J. M. Mendel, “Signal processing with higher-order spectra,” IEEE Signal Processing Magazine, pp. 10–37, July 1993. Google Scholar
- D. L. Harnett and J. L. Murphy, Introductary Statistical Analysis. California: Addison-Wesley, 1975. Google Scholar
- L. Blank, Statistical Procedures for Engineering, Management, and Science. New York: McGraw-Hill, 1980. Google Scholar
- S. Ghahramani, Fundamentals of Probability. New Jersey: Prentice-Hall, 2000. Google Scholar
- O. Kempthorne and L. Folks, Probability, Statistics, and Data Analysis. Iowa: Iowa State Unitversity Press, 1971. MATHGoogle Scholar
- C. W. Therrien, Discrete Random Signals and Statistical Signal Processing. New Jersey: Prentice-Hall, 1992. MATHGoogle Scholar
- N. R. Goodman, “Statistical analysis based on a certain multivariate complex Gaussian distribution (an introduction),” Annals of Math. Statistics, pp. 152–177, 1963. Google Scholar
- S. Haykin, Blind Deconvolution. New Jersey: Prentice-Hall, 1994. Google Scholar
- T. Chonavel, Statistical Signal Processing: Modelling and Estimation. London: Springer-Verlag, 2002, translated by J. Ormrod. MATHGoogle Scholar
- G. L. Wise, “A cautionary aspect of stationary random processes,” IEEE Trans. Circuits and Systems, vol. 38, no. 11, pp. 1409–1410, Nov. 1991. ArticleGoogle Scholar
- J. G. Proakis, Digital Communications, 4th ed. New York: McGraw-Hill, 2001. Google Scholar
- S. M. Kay, Modern Spectral Estimation: Theory and Application. New Jersey: Prentice-Hall, 1988. MATHGoogle Scholar
- S. Haykin, Adaptive Filter Theory. New Jersey: Prentice-Hall, 1996. Google Scholar
- C. L. Nikias and M. R. Raghuveer, “Bispectrum estimation: A digital signal processing framework,” Proc. IEEE, vol. 75, no. 7, pp. 869–891, July 1987. ArticleGoogle Scholar
- D. Hatzinakos, Higher-order Spectral (H.O.S.) Analysis, in Stochastic Techniques in Digital Signal Processing Systems, Part 2 of 2, C.T. Leondes, ed. San Diego: Academic Press, 1994. Google Scholar
- D. R. Brillinger, “Some history of the study of higher-order moments and spectra,” in Proc. IEEE Workshop on Higher-Order Spectral Analysis, Vail, Colorado, June 28–30, 1989, pp. 41–45. Google Scholar
- B. Porat, Digital Processing of Random Signals: Theory and Methods. New Jersey: Prentice-Hall, 1994. Google Scholar
- G. B. Giannakis and M. K. Tsatsanis, “HOS or SOS for parametric modeling?” Proc. IEEE, pp. 3097–3100, 1991. Google Scholar
- A. Swami, G. B. Giannakis, G. Zhou, and L. Srinivas, Bibliography on Higher Order Statistics. Virginia: University of Virginia, Jan. 1995. Google Scholar
- P. A. Delaney and D. O. Walsh, “A bibliography of higher-order spectra and cumulants,” IEEE Signal Processing Magazine, pp. 61–70, July 1994. Google Scholar
- W. A. Gardner and L. E. Franks, “Characterization of cyclostationary random signal processes,” IEEE Trans. Information Theory, vol. IT-21, no. 1, pp. 4–14, Jan. 1975. ArticleGoogle Scholar
- W. A. Gardner, Cyclostationarity in Communications and Signal Processing. New York: IEEE Press, 1994. MATHGoogle Scholar
- H. Meyr, M. Moeneclaey, and S. A. Fechtel, Digital Communication Receivers: Synchronization, Channel Estimation, and Signal Processing. New York: John Wiley & Sons, 1998. Google Scholar
- W. A. Gardner, “Exploitation of spectral redundancy in cyclostationary signals,” IEEE Signal Processing Magazine, pp. 14–36, Apr. 1991. Google Scholar
- G. B. Giannakis, “Linear cyclic correlation approaches for blind identification of FIR channels,” in Proc. IEEE 28th Asilomar Conference on Signals, Systems, and Computers, 1995, pp. 420–424. Google Scholar
- W. A. Gardner and C. M. Spooner, Cyclostationary Signal Processing, in Stochastic Techniques in Digital Signal Processing Systems, Part 2 of 2, C.T. Leondes, ed. San Diego: Academic Press, 1994. Google Scholar
- C. M. Spooner, Higher-order Statistics for Nonlinear Processing of Cyclostationary Signals, A Chapter in Cyclostationarity in Communications and Signal Processing, W.A. Gardner, ed. New York: IEEE Press, 1994. Google Scholar
- R. L. Freeman, “Bits, symbols, bauds, and bandwidth,” IEEE Communications Magazine, pp. 96–99, Apr. 1998. Google Scholar
- P. P. Vaidyanathan, Multirate Systems and Filter Banks. New Jersey: Prentice-Hall, 1993. MATHGoogle Scholar
- L. Tong, G. Xu, and T. Kailath, “Blind identification and equalization of multipath channels,” in Proc. IEEE International Conference on Communications, Chicago, June 1992, pp. 1513–1517. Google Scholar
- L. Tong, G. Xu, and T. Kailath, “A new approach to blind identification and equalization of multipath channels,” in Proc. IEEE 25th Asilomar Conference on Signals, Systems, and Computers, Pacific Grove, California, Nov. 1991, pp. 856–860. Google Scholar
- Z. Ding and Y. Li, “Channel identification using second order cyclic statistics,” in Proc. IEEE 26th Asilomar Conference on Signals, Systems, and Computers, Pacific Grove, California, Oct. 1992, pp. 334–338. Google Scholar
- L. Tong, G. Xu, and T. Kailath, “Fast blind equalization via antenna arrays,” in Proc. IEEE International Conference on Acoustics, Speech, and Signal Processing, vol. 4, 1993, pp. 272–275. ArticleGoogle Scholar
- L. Izzo, A. Napolitano, and L. Paura, Cyclostationary-exploiting Methods for Multipath-channel Identification, A Chapter in Cyclostationarity in Communications and Signal Processing, W. A. Gardner ed. New York: IEEE Press, 1994. Google Scholar
- Z. Ding, Blind Channel Identification and Equalization Using Spectral Correlation Measurements, Part I: Frequency-domain Analysis, A Chapter in Cyclostationarity in Communications and Signal Processing, W. A. Gardner, ed. New York: IEEE Press, 1994. Google Scholar
- Z. Ding and Y. Li, “On channel identification based on second-order cyclic spectra,” IEEE Trans. Signal Processing, vol. 42, no. 5, pp. 1260–1264, May 1994. ArticleGoogle Scholar
- L. Tong, G. Xu, and T. Kailath, “Blind identification and equalization based on second-order statistics: A time domain approach,” IEEE Trans. Information Theory, vol. 40, no. 2, pp. 340–349, Mar. 1994. ArticleGoogle Scholar
- S. M. Kay, Fundamentals of Statistical Signal Processing: Estimation Theory. New Jersey: Prentice-Hall, 1993. MATHGoogle Scholar
- M. D. Srinath and P. K. Rajasekaran, An Introduction to Statistical Signal Processing with Applications. New York: John Wiley & Sons, 1979. Google Scholar
- H. W. Sorenson, Parameter Estimation: Principles and Problems. New York: Marcel Dekker, 1985. Google Scholar
- H. Stark and J. W. Woods, Probability and Random Processes with Applications to Signal Processing. New Jersey: Prentice-Hall, 2002. Google Scholar
- T. B. Fomby, R. C. Hill, and S. R. Johnson, Advanced Econometric Methods. New York: Springer-Verlag, 1984. MATHGoogle Scholar
- T. K. Moon and W. C. Stirling, Mathematical Methods and Algorithms for Signal Processing. New Jersey: Prentice-Hall, 2000. Google Scholar
Rights and permissions
Copyright information
© 2006 Springer-Verlag London Limited
About this chapter
Cite this chapter
(2006). Fundamentals of Statistical Signal Processing. In: Blind Equalization and System Identification. Springer, London. https://doi.org/10.1007/1-84628-218-7_3
Download citation
- DOI : https://doi.org/10.1007/1-84628-218-7_3
- Publisher Name : Springer, London
- Print ISBN : 978-1-84628-022-1
- Online ISBN : 978-1-84628-218-8
- eBook Packages : EngineeringEngineering (R0)
Anyone you share the following link with will be able to read this content:
Get shareable link
Sorry, a shareable link is not currently available for this article.
Copy to clipboard
Provided by the Springer Nature SharedIt content-sharing initiative